Optimizing Property Underwriting Einstein Discovery Model to Maximize Profit Potential
π Are you tired of leaving money on the table in property underwriting? Imagine having a crystal ball that could predict profitability with uncanny accuracy. That's exactly what Einstein Discovery brings to the table, but are you harnessing its full potential?
In today's cutthroat insurance market, maximizing profit isn't just about writing more policies - it's about writing the right ones. Einstein Discovery, when optimized correctly, can be your secret weapon in identifying those golden opportunities. But here's the catch: many underwriters are barely scratching the surface of what this powerful tool can do. π
In this blog post, we'll dive deep into the world of Einstein Discovery for property underwriting. From preparing your data for peak performance to fine-tuning your model for maximum profitability, we'll guide you through each step of the process. Get ready to revolutionize your underwriting approach and watch your profit potential soar!

Understanding Einstein Discovery for Property Underwriting
What is Einstein Discovery?
Einstein Discovery is a powerful AI-driven analytics tool within the Salesforce platform. It uses machine learning algorithms to analyze vast amounts of data, uncovering hidden patterns and insights that can drive informed decision-making in various business processes, including property underwriting.
Benefits for property underwriting
Einstein Discovery offers several key advantages for property underwriting:
Improved risk assessment
Faster decision-making
Enhanced accuracy in pricing
Data-driven insights for policy customization
Benefit | Description |
---|---|
Risk Assessment | Analyzes historical data to identify risk factors |
Decision Speed | Automates complex calculations for quicker underwriting |
Pricing Accuracy | Uses predictive modeling to optimize premium rates |
Policy Customization | Provides insights for tailoring coverage to specific needs |
Key features and capabilities
Einstein Discovery's capabilities make it an invaluable tool for property underwriters:
Predictive analytics: Forecasts potential outcomes based on historical data
Prescriptive insights: Suggests actions to improve underwriting decisions
Automated model building: Creates and refines models with minimal human intervention
Natural language explanations: Provides easy-to-understand insights for non-technical users
By leveraging these features, property underwriters can make more informed decisions, leading to improved profitability and reduced risk exposure. With Einstein Discovery, underwriters can process large volumes of data quickly, identify subtle patterns that humans might miss, and continuously refine their models for optimal performance.

Preparing Data for Optimal Model Performance
Now that we understand the basics of Einstein Discovery for property underwriting, let's dive into the crucial step of preparing data for optimal model performance. This process is essential for ensuring accurate predictions and maximizing profit potential.
A. Identifying relevant data sources
To build a robust Einstein Discovery model, we need to gather data from various sources:
Internal databases (claims history, policy details)
External data providers (property valuations, crime statistics)
Public records (building permits, zoning information)
Weather data (historical patterns, natural disaster risks)
B. Data cleaning and preprocessing
Once data is collected, it's vital to clean and preprocess it:
Remove duplicates and irrelevant entries
Standardize formats (e.g., dates, addresses)
Handle outliers and anomalies
Normalize numerical values
C. Feature engineering for property underwriting
Feature engineering involves creating new variables that can improve model performance:
Feature | Description | Impact on Underwriting |
---|---|---|
Age of property | Calculated from build date | Assess risk of structural issues |
Claim frequency | Number of claims per year | Predict likelihood of future claims |
Neighborhood risk score | Composite of crime rates and property values | Evaluate overall risk profile |
D. Handling missing or incomplete data
Dealing with missing data is crucial for model accuracy:
Imputation techniques (mean, median, or advanced methods)
Creating "missing" flags as new features
Excluding records with excessive missing data
By meticulously preparing our data, we set the foundation for a high-performing Einstein Discovery model that can accurately assess property risks and maximize profit potential in underwriting decisions.
Building the Einstein Discovery Model
Now that we have prepared our data, let's dive into building the Einstein Discovery model for property underwriting. This crucial step will lay the foundation for maximizing profit potential in our underwriting process.
A. Selecting the target variable
The first step in building our model is choosing the target variable. This is the outcome we want to predict or optimize. In property underwriting, common target variables include:
Profitability
Loss ratio
Premium adequacy
For our model, let's focus on profitability as our target variable.
B. Choosing predictive factors
Next, we'll select the predictive factors that will influence our target variable. These factors should be relevant to property underwriting and have a potential impact on profitability. Here's a table of some key predictive factors:
Predictive Factor | Description | Impact on Profitability |
---|---|---|
Property Location | Geographic area | High |
Building Age | Years since construction | Medium |
Construction Type | Materials used | High |
Claims History | Past insurance claims | High |
Occupancy Type | Residential, commercial, etc. | Medium |
C. Setting up model parameters
With our target variable and predictive factors in place, we'll configure the model parameters. This includes:
Choosing the algorithm type (e.g., regression, classification)
Setting the confidence threshold
Determining the maximum number of variables to consider
Selecting the evaluation metric (e.g., R-squared, RMSE)
D. Training and testing the model
The final step is to train and test our Einstein Discovery model. This involves:
Splitting the data into training and testing sets
Running the model on the training data
Evaluating model performance on the test data
Iterating and refining as necessary
By carefully building our Einstein Discovery model, we set the stage for optimizing property underwriting and maximizing profit potential. In the next section, we'll explore how to fine-tune the model for even greater profitability.

Fine-tuning the Model for Profitability
Now that we've built our Einstein Discovery model for property underwriting, it's time to fine-tune it for maximum profitability. This crucial step ensures that our model not only predicts outcomes accurately but also aligns with our business objectives.
Interpreting model insights
Einstein Discovery provides valuable insights into the factors influencing our underwriting decisions. Let's examine these insights:
Key drivers: Identify the most significant variables affecting profitability
Correlations: Understand relationships between different factors
Anomalies: Detect unusual patterns that may require further investigation
Adjusting thresholds and decision points
Based on our model insights, we can optimize our decision-making process:
Set appropriate risk thresholds
Define cut-off points for automatic approvals or rejections
Establish criteria for manual review of borderline cases
Incorporating business rules and constraints
To ensure our model aligns with our company's policies and regulatory requirements, we need to:
Integrate compliance guidelines
Apply underwriting limits and restrictions
Implement risk appetite parameters
Balancing risk and reward
The key to maximizing profit potential lies in striking the right balance between risk and reward. Consider the following:
Risk Level | Potential Reward | Recommended Action |
---|---|---|
Low | Moderate | Increase exposure |
Medium | High | Optimize pricing |
High | Very High | Selective underwriting |
By fine-tuning our Einstein Discovery model, we can create a powerful tool that not only predicts outcomes but also guides us towards more profitable underwriting decisions. In the next section, we'll explore how to implement this optimized model into our underwriting processes.
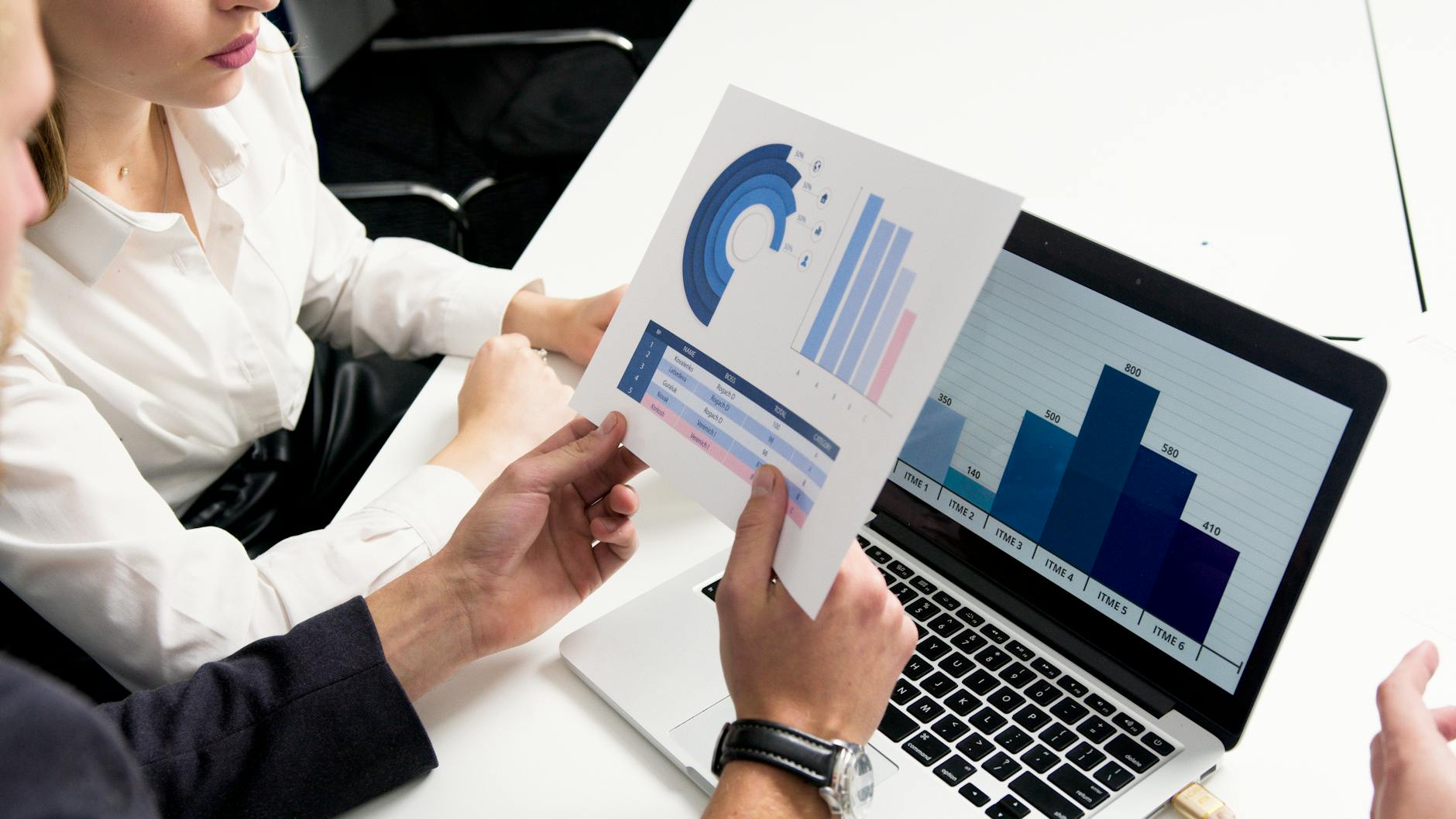
Implementing the Optimized Model in Underwriting Processes
Now that we have fine-tuned our Einstein Discovery model for profitability, it's time to integrate it into our underwriting processes. This critical step ensures that we maximize the potential of our optimized model and drive tangible results.
Integrating with existing systems
Seamless integration is key to successful implementation. Here's a breakdown of the integration process:
Identify touchpoints: Determine where the model will interact with current systems
Data flow mapping: Ensure smooth data transfer between systems
API development: Create robust APIs for real-time model access
Testing: Rigorously test integrations to catch and resolve issues
Training underwriters on model usage
Empowering underwriters with the knowledge to effectively use the model is crucial. Consider the following training approach:
Training Component | Description | Duration |
---|---|---|
Model Overview | Introduction to Einstein Discovery and its benefits | 1 hour |
Hands-on Workshop | Practical exercises using the model | 4 hours |
Q&A Session | Addressing underwriter concerns and queries | 1 hour |
Follow-up Support | Ongoing assistance and resources | Continuous |
Establishing monitoring and feedback loops
To ensure the model continues to perform optimally, implement these monitoring and feedback mechanisms:
Regular performance reviews
Automated alerts for anomalies
Underwriter feedback surveys
Continuous model refinement based on new data and insights
By following these implementation steps, we can effectively leverage our optimized Einstein Discovery model to enhance our property underwriting processes and drive profitability. Next, we'll explore how to measure and maximize the profit potential of our implemented model.

Measuring and Maximizing Profit Potential
Defining key performance indicators (KPIs)
To effectively measure and maximize profit potential in property underwriting, it's crucial to establish the right key performance indicators (KPIs). These metrics will help you gauge the success of your Einstein Discovery model and identify areas for improvement. Some essential KPIs include:
Combined ratio
Loss ratio
Expense ratio
Premium growth
Policy retention rate
Tracking underwriting efficiency and accuracy
Monitoring underwriting efficiency and accuracy is vital for optimizing your Einstein Discovery model. Consider the following metrics:
Turnaround time
Approval rate
Declination rate
Error rate
Underwriter productivity
Metric | Description | Target |
---|---|---|
Turnaround time | Average time to process an application | < 48 hours |
Approval rate | Percentage of approved applications | 70-80% |
Error rate | Percentage of policies with underwriting errors | < 5% |
Analyzing profit margins and loss ratios
Regularly analyze profit margins and loss ratios to ensure your underwriting model is contributing to overall profitability. Key areas to focus on include:
Gross profit margin
Net profit margin
Loss ratio by product line
Loss ratio by geographical region
Identifying opportunities for further optimization
Continuously seek opportunities to enhance your Einstein Discovery model:
Analyze outliers and anomalies in the data
Incorporate new data sources for more accurate predictions
Regularly retrain the model with updated information
Conduct A/B testing to compare model versions
Solicit feedback from underwriters and adjust the model accordingly
By consistently measuring these metrics and identifying areas for improvement, you can maximize the profit potential of your property underwriting Einstein Discovery model.

Overcoming Challenges and Limitations
As we delve into the final section of our discussion on optimizing Einstein Discovery models for property underwriting, it's crucial to address the challenges and limitations that may arise during implementation and ongoing use.
A. Addressing data quality issues
Data quality is the foundation of any successful machine learning model. To ensure optimal performance:
Implement rigorous data validation processes
Regularly audit and cleanse data sources
Establish data governance policies
Data Quality Issue | Solution |
---|---|
Missing values | Use imputation techniques or exclude incomplete records |
Inconsistent formats | Standardize data formats across all sources |
Outliers | Identify and handle outliers through statistical methods |
B. Managing model bias and fairness
Bias in AI models can lead to unfair outcomes and potential legal issues. To mitigate this:
Diversify training data to represent all demographics
Regularly test for bias using multiple fairness metrics
Implement ongoing monitoring and adjustment processes
C. Adapting to changing market conditions
The property market is dynamic, requiring constant model adaptation:
Implement continuous learning mechanisms
Regularly retrain models with fresh data
Monitor model performance against real-world outcomes
D. Ensuring regulatory compliance
Compliance is non-negotiable in the insurance industry:
Stay informed about evolving regulations
Document model decisions and rationale
Implement explainable AI techniques for transparency
By proactively addressing these challenges, insurers can maximize the benefits of Einstein Discovery while minimizing risks and ensuring long-term success in property underwriting.
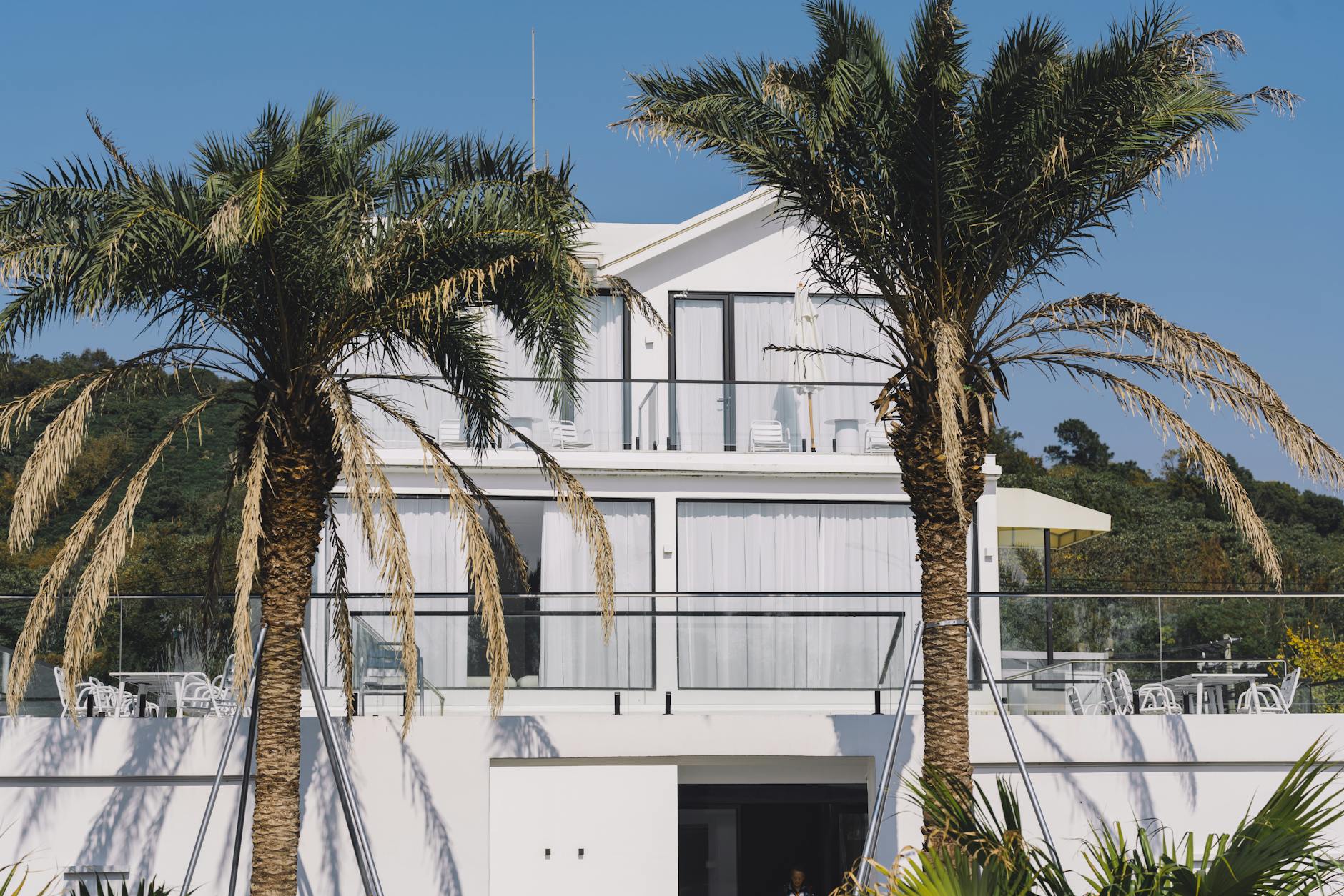
Einstein Discovery has revolutionized property underwriting, offering insurers a powerful tool to maximize profit potential. By leveraging advanced analytics and machine learning, underwriters can make more informed decisions, accurately assess risks, and optimize pricing strategies. The key to success lies in meticulous data preparation, careful model building, and continuous fine-tuning to ensure the model's effectiveness in real-world scenarios.
Implementing an optimized Einstein Discovery model in underwriting processes can significantly enhance profitability. However, it's crucial to remain vigilant in measuring performance, addressing challenges, and adapting to evolving market conditions. By embracing this innovative approach and continuously refining their models, insurers can stay ahead of the competition and unlock new opportunities for growth in the dynamic property insurance landscape.
- Get link
- X
- Other Apps
Comments
Post a Comment